Data AI (60)
The convergence of various technologies is no longer a luxury but a necessity for business success. If you run an eCommerce store, you're already aware of the importance of Search Engine Optimization (SEO) for visibility, traffic, and ultimately, conversions. But have you ever considered how the Internet of Things (IoT) can further enrich your SEO strategy? As disparate as they may seem, IoT and SEO can intersect in fascinating ways to offer significant advantages for your eCommerce business. Let’s delve into the symbiosis between IoT data and eCommerce SEO.
Why Should eCommerce Care About IoT?
IoT can do wonders for the eCommerce sector by enhancing user experience, streamlining operations, and providing unparalleled data insights. Smart homes, wearables, and voice search devices like Amazon's Alexa or Google Home are becoming standard accessories in households, which means that consumers are using IoT for their online shopping needs more than ever.
Integrating IoT Insights into UX Design
IoT data isn't just for SEO; it can also transform your site's User Experience (UX) design. By analyzing real-time user interactions captured by IoT devices, you can refine your site layout and navigation for optimal user engagement and conversion. This seamless blend of IoT insights and UX design elevates your eCommerce platform, making it more responsive to user needs and behaviours.
Unlocking User Behavior Insights
One of the most direct ways IoT can impact your SEO strategy is through enhanced data analytics. Devices like smartwatches or fitness trackers could provide valuable information on consumer habits, routines, and preferences. By integrating this IoT data into your SEO strategy, you can better understand your target audience, refine your keyword focus, and tailor your content to better suit the needs and search intent of potential customers.
Voice Search Optimization
Voice-activated devices are increasingly being used to perform searches and online shopping. As voice search is typically more conversational and question-based, you can use IoT data to understand the common phrases or questions consumers ask these devices. This can help you optimize your product descriptions, FAQs, and even blog posts to align with the natural language used in voice searches.
Local SEO and IoT
The "near me" search query is incredibly popular, thanks in part to IoT devices with geolocation capabilities. People use their smartphones or smartwatches to find the nearest restaurant, gas station, or store. If you have a brick-and-mortar store in addition to your online shop, IoT data can help you target local SEO more effectively by integrating local keywords and ensuring your Google My Business listing is up-to-date.
IoT and Page Experience
With IoT, user experience can go beyond the digital interface to incorporate real-world interactions. For example, a smart fridge could remind users to order more milk, directing them to your online grocery store. If your website isn’t optimized for speed and experience, you could lose these high-intent users. Incorporating IoT insights into your SEO strategy can help you anticipate these needs and optimize your site accordingly.
Real-Time Personalization
IoT devices can collect data in real-time, offering insights into user behaviour that can be immediately acted upon. Imagine someone just completed a workout on their smart treadmill. They might then search for protein shakes or workout gear. With real-time data, you could offer timely discounts or suggestions, personalized to the user's immediate needs, all while improving your SEO through higher user engagement and lower bounce rates.
Wrapping It Up
IoT and SEO may seem like different arenas, but they are more interconnected than you'd think. By adopting a holistic approach that marries the insights from IoT devices with your SEO strategy, you can significantly improve your eCommerce site's performance. From optimizing for voice search and improving local SEO to real-time personalization and superior user experience, the opportunities are endless.
The Internet of Things (IoT) continues to revolutionize industries, and Microsoft Azure IoT is at the forefront of this transformation. With its robust suite of services and features, Azure IoT enables organizations to connect, monitor, and manage their IoT devices and data effectively. In this blog post, we will explore the latest trends and use cases of Azure IoT in 2023, showcasing how it empowers businesses across various sectors.
Edge Computing and AI at the Edge:
As the volume of IoT devices and the need for real-time analytics increases, edge computing has gained significant momentum. Azure IoT enables edge computing by seamlessly extending its capabilities to the edge devices. In 2023, we can expect Azure IoT to further enhance its edge computing offerings, allowing organizations to process and analyze data closer to the source. With AI at the edge, businesses can leverage machine learning algorithms to gain valuable insights and take immediate actions based on real-time data.
Edge Computing and Real-time Analytics:
As IoT deployments scale, the demand for real-time data processing and analytics at the edge has grown. Azure IoT Edge allows organizations to deploy and run cloud workloads directly on IoT devices, enabling quick data analysis and insights at the edge of the network. With edge computing, businesses can reduce latency, enhance security, and make faster, data-driven decisions.
Industrial IoT (IIoT) for Smart Manufacturing:
Azure IoT is poised to play a crucial role in the digital transformation of manufacturing processes. IIoT solutions built on Azure enable manufacturers to connect their machines, collect data, and optimize operations. In 2023, we anticipate Azure IoT to continue empowering smart manufacturing by offering advanced analytics, predictive maintenance, and intelligent supply chain management. By harnessing the power of Azure IoT, manufacturers can reduce downtime, enhance productivity, and achieve greater operational efficiency.
Connected Healthcare:
In the healthcare industry, Azure IoT is revolutionizing patient care and operational efficiency. In 2023, we expect Azure IoT to drive the connected healthcare ecosystem further. IoT-enabled medical devices, remote patient monitoring systems, and real-time data analytics can help healthcare providers deliver personalized care, improve patient outcomes, and optimize resource allocation. Azure IoT's robust security and compliance features ensure that sensitive patient data remains protected throughout the healthcare continuum.
Smart Cities and Sustainable Infrastructure:
As cities strive to become more sustainable and efficient, Azure IoT offers a powerful platform for smart city initiatives. In 2023, Azure IoT is likely to facilitate the deployment of smart sensors, intelligent transportation systems, and efficient energy management solutions. By leveraging Azure IoT, cities can enhance traffic management, reduce carbon emissions, and improve the overall quality of life for their residents.
Retail and Customer Experience:
Azure IoT is transforming the retail landscape by enabling personalized customer experiences, inventory optimization, and real-time supply chain visibility. In 2023, we can expect Azure IoT to continue enhancing the retail industry with innovations such as cashier-less stores, smart shelves, and automated inventory management. By leveraging Azure IoT's capabilities, retailers can gain valuable insights into customer behavior, streamline operations, and deliver superior shopping experiences.
AI and Machine Learning Integration:
Azure IoT integrates seamlessly with Microsoft's powerful artificial intelligence (AI) and machine learning (ML) capabilities. By leveraging Azure IoT and Azure AI services, organizations can gain actionable insights from their IoT data. For example, predictive maintenance algorithms can analyze sensor data to detect equipment failures before they occur, minimizing downtime and optimizing operational efficiency.
Enhanced Security and Device Management:
In an increasingly interconnected world, security is a top priority for IoT deployments. Azure IoT provides robust security features to protect devices, data, and communications. With features like Azure Sphere, organizations can build secure and trustworthy IoT devices, while Azure IoT Hub ensures secure and reliable device-to-cloud and cloud-to-device communication. Additionally, Azure IoT Central simplifies device management, enabling organizations to monitor and manage their IoT devices at scale.
Industry-specific Solutions:
Azure IoT offers industry-specific solutions tailored to the unique needs of various sectors. Whether it's manufacturing, healthcare, retail, or transportation, Azure IoT provides pre-built solutions and accelerators to jumpstart IoT deployments. For example, in manufacturing, Azure IoT helps optimize production processes, monitor equipment performance, and enable predictive maintenance. In healthcare, it enables remote patient monitoring, asset tracking, and patient safety solutions.
Integration with Azure Services:
Azure IoT seamlessly integrates with a wide range of Azure services, creating a comprehensive ecosystem for IoT deployments. Organizations can leverage services like Azure Functions for serverless computing, Azure Stream Analytics for real-time data processing, Azure Cosmos DB for scalable and globally distributed databases, and Azure Logic Apps for workflow automation. This integration enables organizations to build end-to-end IoT solutions with ease.
Conclusion:
In 2023, Azure IoT is set to drive innovation across various sectors, including manufacturing, healthcare, cities, and retail. With its robust suite of services, edge computing capabilities, and AI integration, Azure IoT empowers organizations to harness the full potential of IoT and achieve digital transformation. As businesses embrace the latest trends and leverage the diverse use cases of Azure IoT, they can gain a competitive edge, improve operational efficiency, and unlock new opportunities in the connected world.
About Infysion
We work closely with our clients to help them successfully build and execute their most critical strategies. We work behind-the-scenes with machine manufacturers and industrial SaaS providers, to help them build intelligent solutions around Condition based machine monitoring, analytics-driven Asset management, accurate Failure predictions and end-to-end operations visibility. Since our founding 3 years ago, Infysion has successfully productionised over 20+ industry implementations, that support Energy production, Water & electricity supply monitoring, Wind & Solar farms management, assets monitoring and Healthcare equipment monitoring.
We strive to provide our clients with exceptional software and services that will create a meaningful impact on their bottom line.
Visit our website to learn more about success stories, how we work, Latest Blogs and different services we do offer!
Cloud-based motor monitoring as a service is revolutionizing the way industries manage and maintain their critical assets. By leveraging the power of the cloud, organizations can remotely monitor motors, analyze performance data, and predict potential failures. However, as this technology continues to evolve, several challenges emerge that need to be addressed for successful implementation and operation. In this blog post, we will explore the top challenges faced in cloud-based motor monitoring as a service in 2023.
Data Security and Privacy:
One of the primary concerns in cloud-based motor monitoring is ensuring the security and privacy of sensitive data. As motor data is transmitted and stored in the cloud, there is a need for robust encryption, authentication, and access control mechanisms. In 2023, organizations will face the challenge of implementing comprehensive data security measures to protect against unauthorized access, data breaches, and potential cyber threats. Compliance with data privacy regulations, such as GDPR or CCPA, adds an additional layer of complexity to this challenge.
Connectivity and Network Reliability:
For effective motor monitoring, a reliable and secure network connection is crucial. In remote or industrial environments, ensuring continuous connectivity can be challenging. Factors such as signal strength, network coverage, and bandwidth limitations need to be addressed to enable real-time data transmission and analysis. Organizations in 2023 will need to deploy robust networking infrastructure, explore alternative connectivity options like satellite or cellular networks, and implement redundancy measures to mitigate the risk of network disruptions.
Scalability and Data Management:
Cloud-based motor monitoring generates vast amounts of data that need to be efficiently processed, stored, and analyzed. In 2023, as the number of monitored motors increases, organizations will face challenges in scaling their data management infrastructure. They will need to ensure that their cloud-based systems can handle the growing volume of data, implement efficient data storage and retrieval mechanisms, and utilize advanced analytics and machine learning techniques to extract meaningful insights from the data.
Integration with Existing Systems:
Integrating cloud-based motor monitoring systems with existing infrastructure and software can pose significant challenges. In 2023, organizations will need to ensure seamless integration with their existing enterprise resource planning (ERP), maintenance management, and asset management systems. This includes establishing data pipelines, defining standardized protocols, and implementing interoperability between different systems. Compatibility with various motor types, brands, and communication protocols also adds complexity to the integration process.
Cost and Return on Investment:
While cloud-based motor monitoring offers numerous benefits, organizations must carefully evaluate the cost implications and expected return on investment (ROI). Implementing and maintaining the necessary hardware, software, and cloud infrastructure can incur significant expenses. Organizations in 2023 will face the challenge of assessing the financial viability of cloud-based motor monitoring, considering factors such as deployment costs, ongoing operational expenses, and the potential savings achieved through improved motor performance, reduced downtime, and optimized maintenance schedules.
Connectivity and Reliability:
Cloud-based motor monitoring relies heavily on stable and reliable internet connectivity. However, in certain remote locations or industrial settings, maintaining a consistent connection can be challenging. The availability of high-speed internet, network outages, or intermittent connections may impact real-time monitoring and timely data transmission. Service providers will need to address connectivity issues to ensure uninterrupted monitoring and minimize potential disruptions.
Scalability and Performance:
As the number of monitored motors increases, scalability and performance become critical challenges. Service providers must design their cloud infrastructure to handle the growing volume of data generated by motor sensors. Ensuring real-time data processing, analytics, and insights at scale will be vital to meet the demands of large-scale motor monitoring deployments. Continuous optimization and proactive capacity planning will be necessary to maintain optimal performance levels.
Integration with Legacy Systems:
Integrating cloud-based motor monitoring with existing legacy systems can be a complex undertaking. Many organizations have legacy equipment or infrastructure that may not be inherently compatible with cloud-based solutions. The challenge lies in seamlessly integrating these disparate systems to enable data exchange and unified monitoring. Service providers need to offer flexible integration options, standardized protocols, and compatibility with a wide range of motor types and manufacturers.
Data Analytics and Actionable Insights:
Collecting data from motor sensors is only the first step. The real value lies in extracting actionable insights from this data to enable predictive maintenance, identify performance trends, and optimize motor operations. Service providers must develop advanced analytics capabilities that can process large volumes of motor data and provide meaningful insights in a user-friendly format. The challenge is to offer intuitive dashboards, anomaly detection, and predictive analytics that empower users to make data-driven decisions effectively.
Conclusion:
Cloud-based motor monitoring as a service offers tremendous potential for organizations seeking to optimize motor performance and maintenance. However, in 2023, several challenges need to be addressed to ensure its successful implementation. From data security and connectivity issues to scalability, integration, and advanced analytics, service providers must actively tackle these challenges to unlock the full benefits of cloud-based motor monitoring. By doing so, organizations can enhance operational efficiency, extend motor lifespan, and reduce costly downtime in the ever-evolving landscape of motor-driven industries.
Data is a critical resource in IoT that enables organizations to gain insights into their operations, optimize processes, and improve customer experience. It is important to understand the cost of managing and processing data, as it can be significant. Too often, organizations have more data than they know how to effectively use. Here are some of the major areas of costs:
First, data storage is a major cost. IoT devices generate large amounts of data, and this data needs to be stored in a secure and reliable way. Storing data in the cloud or on remote servers can be expensive, as it requires a robust and scalable infrastructure to support the large amounts of data generated by IoT devices. Additionally, data must be backed up to ensure data integrity and security, which adds to the cost.
Second, data processing and analysis require significant computational resources. Processing large amounts of data generated by IoT devices requires high-performance hardware and software, which can be expensive to acquire and maintain. Additionally, hiring data scientists and other experts to interpret and analyze the data adds to the cost.
Third, data transmission over networks can be costly. IoT devices generate data that needs to be transmitted over networks to be stored and processed. Depending on the location of IoT devices and the network infrastructure, the cost of network connectivity can vary widely.
Finally, data security is a major concern in IoT, and implementing robust security measures can add to the cost. This includes implementing encryption protocols to ensure data confidentiality, as well as implementing measures to prevent unauthorized access to IoT devices and data.
Managing and processing data requires significant resources, including storage, processing and analysis, network connectivity, and security. While data is a valuable resource that can provide significant value, the cost of managing and processing data must be carefully evaluated to ensure that the benefits outweigh the expenses.
Adaptive systems and models at runtime refer to the ability of a system or model to dynamically adjust its behavior or parameters based on changing conditions and feedback during runtime. This allows the system or model to better adapt to its environment, improve its performance, and enhance its overall effectiveness.
Some technical details about adaptive systems and models at runtime include:
Feedback loops: Adaptive systems and models rely on feedback loops to gather data and adjust their behavior. These feedback loops can be either explicit or implicit, and they typically involve collecting data from sensors or other sources, analyzing the data, and using it to make decisions about how to adjust the system or model.
Machine learning algorithms: Machine learning algorithms are often used in adaptive systems and models to analyze feedback data and make predictions about future behavior. These algorithms can be supervised, unsupervised, or reinforcement learning-based, depending on the type of feedback data available and the desired outcomes.
Parameter tuning: In adaptive systems and models, parameters are often adjusted dynamically to optimize performance. This can involve changing things like thresholds, time constants, or weighting factors based on feedback data.
Self-organizing systems: Some adaptive systems and models are designed to be self-organizing, meaning that they can reconfigure themselves in response to changing conditions without requiring external input. Self-organizing systems typically use decentralized decision-making and distributed control to achieve their goals.
Context awareness: Adaptive systems and models often incorporate context awareness, meaning that they can adapt their behavior based on situational factors like time of day, location, or user preferences. This requires the use of sensors and other data sources to gather information about the environment in real-time.
Overall, adaptive systems and models at runtime are complex and dynamic, requiring sophisticated algorithms and techniques to function effectively. However, the benefits of these systems can be significant, including improved performance, increased flexibility, and better overall outcomes.
With the advent of the Internet of Things, Big Data is becoming more and more important. After all, when you have devices that are constantly collecting data, you need somewhere to store it all. But the Internet of Things is not just changing the way we store data; it’s changing the way we collect and use it as well. In this blog post, we will explore how the Internet of Things is transforming Big Data. From new data sources to new ways of analyzing data, the Internet of Things is changing the Big Data landscape in a big way.
How is the Internet of Things transforming Big Data?
The Internet of Things is transforming Big Data in a number of ways. One way is by making it possible to collect more data than ever before. This is because devices that are connected to the Internet can generate a huge amount of data. This data can be used to help businesses and organizations make better decisions.
Another way the Internet of Things is transforming Big Data is by making it easier to process and analyze this data. This is because there are now many tools and technologies that can help with this. One example is machine learning, which can be used to find patterns in data.
The Internet of Things is also changing the way we think about Big Data. This is because it’s not just about collecting large amounts of data – it’s also about understanding how this data can be used to improve our lives and businesses.
The Benefits of the Internet of Things for Big Data
- The internet of things offers a number of benefits for big data.
- It allows for a greater volume of data to be collected and stored.
- Also, it provides a more diverse range of data types, which can be used to create more accurate and comprehensive models.
- It enables real-time data collection and analysis, which can help organizations make better decisions and take action more quickly.
- It can improve the accuracy of predictions by using historical data to train predictive models.
- Finally, the internet of things can help reduce the cost of storing and processing big data.
The Challenges of the Internet of Things for Big Data
The internet of things is transforming big data in a number of ways. One challenge is the sheer volume of data that is generated by devices and sensors. Another challenge is the variety of data formats, which can make it difficult to derive insights. Additionally, the real-time nature of data from the internet of things presents challenges for traditional big data infrastructure.
Conclusion
The Internet of Things is bringing a new level of connectivity to the world, and with it, a huge influx of data. This data is transforming how businesses operate, giving them new insights into their customers and operations.
The Internet of Things is also changing how we interact with the world around us, making our lives more convenient and efficient. With so much potential, it's no wonder that the Internet of Things is one of the most talked-about topics in the tech world today.
-
Univariate: considering a single variable
-
Multivariate: considering multiple variables
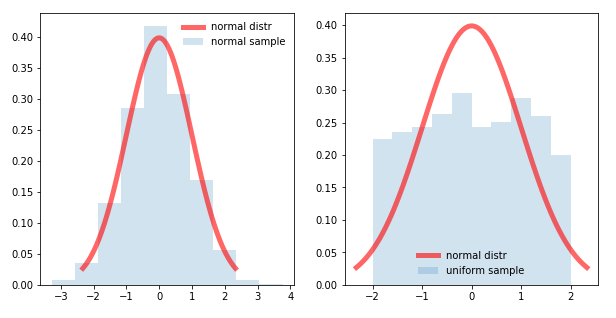

For object detection projects, labeling your images with their corresponding bounding boxes and names is a tedious and time-consuming task, often requiring a human to label each image by hand. The Edge Impulse Studio has already dramatically decreased the amount of time it takes to get from raw images to a fully labeled dataset with the Data Acquisition Labeling Queue feature directly in your web browser. To make this process even faster, the Edge Impulse Studio is getting a new feature: AI-Assisted Labeling.
Automatically label common objects with YOLOv5.
To get started, create a “Classify multiple objects” images project via the Edge Impulse Studio new project wizard or open your existing object detection project. Upload your object detection images to your Edge Impulse project’s training and testing sets. Then, from the Data Acquisition tab, select “Labeling queue.”
1. Using YOLOv5
By utilizing an existing library of pre-trained object detection models from YOLOv5 (trained with the COCO dataset), common objects in your images can quickly be identified and labeled in seconds without needing to write any code!
To label your objects with YOLOv5 classification, click the Label suggestions dropdown and select “Classify using YOLOv5.” If your object is more specific than what is auto-labeled by YOLOv5, e.g. “coffee” instead of the generic “cup” class, you can modify the auto-labels to the left of your image. These modifications will automatically apply to future images in your labeling queue.
Click Save labels to move on to your next raw image, and see your fully labeled dataset ready for training in minutes!
2. Using your own model
You can also use your own trained model to predict and label your new images. From an existing (trained) Edge Impulse object detection project, upload new unlabeled images from the Data Acquisition tab. Then, from the “Labeling queue”, click the Label suggestions dropdown and select “Classify using <your project name>”:
You can also upload a few samples to a new object detection project, train a model, then upload more samples to the Data Acquisition tab and use the AI-Assisted Labeling feature for the rest of your dataset. Classifying using your own trained model is especially useful for objects that are not in YOLOv5, such as industrial objects, etc.
Click Save labels to move on to your next raw image, and see your fully labeled dataset ready for training in minutes using your own pre-trained model!
3. Using object tracking
If you have objects that are a similar size or common between images, you can also track your objects between frames within the Edge Impulse Labeling Queue, reducing the amount of time needed to re-label and re-draw bounding boxes over your entire dataset.
Draw your bounding boxes and label your images, then, after clicking Save labels, the objects will be tracked from frame to frame:
Track and auto-label your objects between frames.
Now that your object detection project contains a fully labeled dataset, learn how to train and deploy your model to your edge device: check out our tutorial!
Originally posted on the Edge Impulse blog by Jenny Plunkett - Senior Developer Relations Engineer.
Connected devices are emerging as a modern way for grocers to decrease food spoilage and energy waste losses. With bottom-line advantages, it is not surprising to experience some of the biggest business giants are putting internet of things (IoT) techniques to work and enhance the operating results.
According to Talk Business, Walmart uses IoT for different tasks like tracking food temperature, equipment energy outputs, etc. IoT apps help monitor refrigeration units for several products such as milk cold, ice cream, etc. It reports back to a support team if sensors have intimate equipment difficulties fixed without serious malfunctions and minimal downtime.
IoT solutions are used broadly during Walmart's massive store footprint. The connected devices send a total of 1.5 billion messages each day. Throughout the grocery business, IoT is leveraged to enhance food safety and decrease excessive energy consumption. IoT solutions allow food retailers to reduce food spoilage by 40% and experience a net energy saving of 30%.
Image: (Source)
It was forecasted that in 2018 grocers lose around $70 million per year due to food spoilage. However, large chains are losing hundreds of millions due to the same. Hence most grocers have started implementing sustainability-focused IoT technology to avoid wastage and increase their business profit to a great extent.
Image: (Source)
Explore How IoT Helps to Offer Safer Shopping Experience to Shoppers
People prepare to stock up food in preparation; however, there are numerous challenges that the pandemic raised in front of retails. But more retail trends are an answer to all the challenges, beginning from product moving to stock and much more.
It also helps to ensure safe and healthy deliveries to customers' doorsteps, especially whenever they need it. Harvard study shows that grocery shopping is a high-risk activity than traveling on an airplane during COVID 19 pandemic. With COID 19 pandemic raging, retailer stores need to provide an efficient shopping experience; they must look for ways that help them overcome exposure and the risk of infection as customers venture to the store for food.
Image: (Source)
Most grocers turn towards modern technology such as IoT to help retailers or supermarkets offer safe service and meet the bottom lines. By placing internet of things devices throughout the store, smart grocery carts, baskets, etc., grocers can help make experiences more efficient and safer. Let's check how IoT is helping retailer businesses to overcome today's challenging scenarios and stop food spoilage.
Smart Stock Monitoring
Retailers keep warehouses full of goods to ensure that they don't run when there is high demand. And by integrating IoT-enabled sensors, retailers can easily detect weight on sleeves at warehouses and stores. It also helps them determine popular item lists; keeping track of items helps retailers restock them and prevent overstocking a particular product.
Guaranteeing Timely Deliveries
The report shows that 66% of customers anticipate they will increase online shopping in 2020. Undoubtedly online shopping is a new norm these days; most people prefer to order their daily essentials using a grocery delivery mobile app. However, it becomes vital for brands to ensure timely delivery. It's a critical factor, especially when it comes to customer satisfaction, especially when there is a lack of traditional consumer engagement like a friendly salesperson.
And by integrating IoT-enabled devices into containers and shipments, retailers can quickly obtain insight into shipments. They can even track real-time updates to keep their customers up to date on the approximate delivery time. It's critical, especially when you want to achieve excellent customer experiences in the eCommerce market.
However, data collected using IoT-enabled devices can help you drive the supply chain effectively by empowering retailers with root optimization for ensuring fast delivery. The IoT can play a crucial role, especially when it comes to recognizing warehouse delays. It also helps to optimize delivery operations for better and quicker service.
Manage Store Capacity
With the new COVID 19 safety guidelines to follow, IoT helps retailers ensure their customer's safety by supporting social distancing rules. For example, retailers can place IoT sensors at the entrance and exit to efficiently monitor traffic and grocery carts. The sensors provide accurate and up-to-date details. Details enable retailers to efficiently operate capacity, ensuring safety, and eliminates the need for store "bouncers" at exit and entrance.
Contact Monitoring
Retailers can offer a safe and unique shopping experience by benefiting from IoT. It helps them with contact monitoring and social distancing as well. Retailers can provide shoppers with IoT-enabled wearables paired with the shopper's mobile phone through their branded app. It helps shoppers detect whether they are too close to another shopper and report them through their phone and record the incident.
Combating COVID 19: How IoT is Helping Retailers?
Preventing food spoilage, saving energy, and reducing waste are good practices for grocery stores helping them to increase their profit margin. Due to the coronavirus pandemic, digital resilience has boosted drastically.
Many brands and retailers, however, put a pause on initiatives during COVID 19. But to ensure their survival and profit margins, they need to start with new strategies and techniques. Check few IoT use cases that retailers are considering these days:
Video Analytics
Although supermarkets have practiced video surveillance technologies for the last many years, some brands are repurposing these systems to enhance their inventory management practices. Cameras would monitor consumer behaviors and help retailers to prevent theft.
As the customer's purchase preference changes constantly, it becomes essential for grocery stores to start stocking more perishable goods. A 2018 survey shows that more than 60% of retailers integrate refrigerators to store fresh products at their stores and meet customers' growing demand.
And by monitoring customers' purchasing patterns, grocery stores can gauge how much extra produce they need to acquire and how unexpected surges and falls in the market will affect their margins.
Autonomous Cleaning Robots
To promote social distancing, grocery stores are taking all essential precautions. They have implemented a rigorous cleaning schedule to reduce the risk of COVID 19 spread. Retailers are focusing on sanitizing and disinfecting frequent touch surfaces using autonomous cleaning robots.
Robots can be controlled using IoT-based devices and help to sanitize various parts, including doors, shopping carts, countertops, etc. All these tasks demand a reasonable amount of time and employees' attention as well. But performing functions with the help of autonomous cleaning robots can help retailers save their employees time and energy.
Contactless Checkout
Contactless checkout has become increasingly popular over the few years. It has helped supermarkets reduce the requirement for cashier dedication to increase the customers' shopping speed. During the COVID 19 pandemic, the self-service environments have allowed customers a way to acquire essential food, cleaning products, and other day-to-day essentials without having to communicate with supermarket staff directly.
What's Next for Smart Supermarkets?
IoT technologies help supermarkets to tackle new challenges efficiently. Most stores know that they receive products from a different location, which went to a distribution center, making them lose traceability. But with IoT integration, it has become easier for them to track every business activity and provide an internet of shopping experience to customers.
Modern IoT technology makes it possible for grocery stores to track every activity at each stage. It becomes crucial for the health and safety of your customers, while it helps to handle top purchase priorities. It helps grocery stores exist and maintain healthy purchasing trends. IoT initiatives provide the shopping 2.0 infrastructure like smart shelves, carts, cashless, and other options that change the primary service experience.
The head is surely the most complex group of organs in the human body, but also the most delicate. The assessment and prevention of risks in the workplace remains the first priority approach to avoid accidents or reduce the number of serious injuries to the head. This is why wearing a hard hat in an industrial working environment is often required by law and helps to avoid serious accidents.
This article will give you an overview of how to detect that the wearing of a helmet is well respected by all workers using a machine learning object detection model.
For this project, we have been using:
- Edge Impulse Studi to acquire some custom data, visualize the data, train the machine learning model and validate the inference results.
- Part of this public dataset from Roboflow, where the images containing the smallest bounding boxes has been removed.
- Part of the Flicker-Faces-HQ (FFHQ) (under Creative Commons BY 2.0 license) to rebalance the classes in our dataset.
- Google Colab to convert the Yolo v5 PyTorch format from the public dataset to Edge Impulse Ingestion format.
- A Rasberry Pi, NVIDIA Jetson Nano or with any Intel-based Macbooks to deploy the inference model.
Before we get started, here are some insights of the benefits / drawbacks of using a public dataset versus collecting your own.
Using a public dataset is a nice-to-have to start developing your application quickly, validate your idea and check the first results. But we often get disappointed with the results when testing on your own data and in real conditions. As such, for very specific applications, you might spend much more time trying to tweak an open dataset rather than collecting your own. Also, remember to always make sure that the license suits your needs when using a dataset you found online.
On the other hand, collecting your own dataset can take a lot of time, it is a repetitive task and most of the time annoying. But, it gives the possibility to collect data that will be as close as possible to your real life application, with the same lighting conditions, the same camera or the same angle for example. Therefore, your accuracy in your real conditions will be much higher.
Using only custom data can indeed work well in your environment but it might not give the same accuracy in another environment, thus generalization is harder.
The dataset which has been used for this project is a mix of open data, supplemented by custom data.
First iteration, using only the public datasets
At first, we tried to train our model only using a small portion of this public dataset: 176 items in the training set and 57 items in the test set where we took only images containing a bounding box bigger than 130 pixels, we will see later why.
If you go through the public dataset, you can see that the entire dataset is strongly missing some “head” data samples. The dataset is therefore considered as imbalanced.
Several techniques exist to rebalance a dataset, here, we will add new images from Flicker-Faces-HQ (FFHQ). These images do not have bounding boxes but drawing them can be done easily in the Edge Impulse Studio. You can directly import them using the uploader portal. Once your data has been uploaded, just draw boxes around the heads and give it a label as below:
Now that the dataset is more balanced, with both images and bounding boxes of hard hats and heads, we can create an impulse, which is a mix of digital signal processing (DSP) blocks and training blocks:
In this particular object detection use case, the DSP block will resize an image to fit the 320x320 pixels needed for the training block and extract meaningful features for the Neural Network. Although the extracted features don’t show a clear separation between the classes, we can start distinguishing some clusters:
To train the model, we selected the Object Detection training block, which fine tunes a pre-trained object detection model on your data. It gives a good performance even with relatively small image datasets. This object detection learning block relies on MobileNetV2 SSD FPN-Lite 320x320.
According to Daniel Situnayake, co-author of the TinyML book and founding TinyML engineer at Edge Impulse, this model “works much better for larger objects—if the object takes up more space in the frame it’s more likely to be correctly classified.” This has been one of the reason why we got rid of the images containing the smallest bounding boxes in our import script.
After training the model, we obtained a 61.6% accuracy on the training set and 57% accuracy on the testing set. You also might note a huge accuracy difference between the quantized version and the float32 version. However, during the linux deployment, the default model uses the unoptimized version. We will then focus on the float32 version only in this article.
This accuracy is not satisfying, and it tends to have trouble detecting the right objects in real conditions:
Second iteration, adding custom data
On the second iteration of this project, we have gone through the process of collecting some of our own data. A very useful and handy way to collect some custom data is using our mobile phone. You can also perform this step with the same camera you will be using in your factory or your construction site, this will be even closer to the real condition and therefore work best with your use case. In our case, we have been using a white hard hat when collecting data. For example, if your company uses yellow ones, consider collecting your data with the same hard hats.
Once the data has been acquired, go through the labeling process again and retrain your model.
We obtain a model that is slightly more accurate when looking at the training performances. However, in real conditions, the model works far better than the previous one.
Finally, to deploy your model on yourA Rasberry Pi, NVIDIA Jetson Nano or your Intel-based Macbook, just follow the instructions provided in the links. The command line interface `edge-impulse-linux-runner` will create a lightweight web interface where you can see the results.
Note that the inference is run locally and you do not need any internet connection to detect your objects. Last but not least, the trained models and the inference SDK are open source. You can use it, modify it and integrate it to a broader application matching specifically to your needs such as stopping a machine when a head is detected for more than 10 seconds.
This project has been publicly released, feel free to have a look at it on Edge Impulse studio, clone the project and go through every steps to get a better understanding: https://studio.edgeimpulse.com/public/34898/latest
The essence of this use case is, Edge Impulse allows with very little effort to develop industry grade solutions in the health and safety context. Now this can be embedded in bigger industrial control and automation systems with a consistent and stringent focus on machine operations linked to H&S complaint measures. Pre-training models, which later can be easily retrained in the final industrial context as a step of “calibration,” makes this a customizable solution for your next project.
Originally posted on the Edge Impulse blog by Louis Moreau - User Success Engineer at Edge Impulse & Mihajlo Raljic - Sales EMEA at Edge Impulse
By Ricardo Buranello
What Is the Concept of a Virtual Factory?
For a decade, the first Friday in October has been designated as National Manufacturing Day. This day begins a month-long events schedule at manufacturing companies nationwide to attract talent to modern manufacturing careers.
For some period, manufacturing went out of fashion. Young tech talents preferred software and financial services career opportunities. This preference has changed in recent years. The advent of digital technologies and robotization brought some glamour back.
The connected factory is democratizing another innovation — the virtual factory. Without critical asset connection at the IoT edge, the virtual factory couldn’t have been realized by anything other than brand-new factories and technology implementations.
There are technologies that enable decades-old assets to communicate. Such technologies allow us to join machine data with physical environment and operational conditions data. Benefits of virtual factory technologies like digital twin are within reach for greenfield and legacy implementations.
Digital twin technologies can be used for predictive maintenance and scenario planning analysis. At its core, the digital twin is about access to real-time operational data to predict and manage the asset’s life cycle. It leverages relevant life cycle management information inside and outside the factory. The possibilities of bringing various data types together for advanced analysis are promising.
I used to see a distinction between IoT-enabled greenfield technology in new factories and legacy technology in older ones. Data flowed seamlessly from IoT-enabled machines to enterprise systems or the cloud for advanced analytics in new factories’ connected assets. In older factories, while data wanted to move to the enterprise systems or the cloud, it hit countless walls. Innovative factories were creating IoT technologies in proof of concepts (POCs) on legacy equipment, but this wasn’t the norm.
No matter the age of the factory or equipment, everything looks alike. When manufacturing companies invest in machines, the expectation is this asset will be used for a decade or more. We had to invent something inclusive to new and legacy machines and systems.
We had to create something to allow decades-old equipment from diverse brands and types (PLCs, CNCs, robots, etc.) to communicate with one another. We had to think in terms of how to make legacy machines to talk to legacy systems. Connecting was not enough. We had to make it accessible for experienced developers and technicians not specialized in systems integration.
If plant managers and leaders have clear and consumable data, they can use it for analysis and measurement. Surfacing and routing data has enabled innovative use cases in processes controlled by aged equipment. Prescriptive and predictive maintenance reduce downtime and allow access to data. This access enables remote operation and improved safety on the plant floor. Each line flows better, improving supply chain orchestration and worker productivity.
Open protocols aren’t optimized for connecting to each machine. You need tools and optimized drivers to connect to the machines, cut latency time and get the data to where it needs to be in the appropriate format to save costs. These tools include:
- Machine data collection
- Data transformation and visualization
- Device management
- Edge logic
- Embedded security
- Enterprise integration
Plants are trying to get and use data to improve overall equipment effectiveness. OEE applications can calculate how many good and bad parts were produced compared to the machine’s capacity. This analysis can go much deeper. Factories can visualize how the machine works down to sub-processes. They can synchronize each movement to the millisecond and change timing to increase operational efficiency.
The technology is here. It is mature. It’s no longer a question of whether you want to use it — you have it to get to what’s next. I think this makes it a fascinating time for smart manufacturing.
Originally posted here.
By Tony Pisani
For midstream oil and gas operators, data flow can be as important as product flow. The operator’s job is to safely move oil and natural gas from its extraction point (upstream), to where it’s converted to fuels (midstream), to customer delivery locations (downstream). During this process, pump stations, meter stations, storage sites, interconnection points, and block valves generate a substantial volume and variety of data that can lead to increased efficiency and safety.
“Just one pipeline pump station might have 6 Programmable Logic Controllers (PLCs), 12 flow computers, and 30 field instruments, and each one is a source of valuable operational information,” said Mike Walden, IT and SCADA Director for New Frontier Technologies, a Cisco IoT Design-In Partner that implements OT and IT systems for industrial applications. Until recently, data collection from pipelines was so expensive that most operators only collected the bare minimum data required to comply with industry regulations. That data included pump discharge pressure, for instance, but not pump bearing temperature, which helps predict future equipment failures.
A turnkey solution to modernize midstream operations
Now midstream operators are modernizing their pipelines with Industrial Internet of Things (IIoT) solutions. Cisco and New Frontier Technologies have teamed up to offer a solution combining the Cisco 1100 Series Industrial Integrated Services Router, Cisco Edge Intelligence, and New Frontier’s know-how. Deployed at edge locations like pump stations, the solution extracts data from pipeline equipment and is sent via legacy protocols, transforming data at the edge to a format that analytics and other enterprise applications understand. The transformation also minimizes bandwidth usage.
Mike Walden views the Cisco IR1101 as a game-changer for midstream operators. He shared with me that “Before the Cisco IR1101, our customers needed four separate devices to transmit edge data to a cloud server—a router at the pump station, an edge device to do protocol conversion from the old to the new, a network switch, and maybe a firewall to encrypt messages…With the Cisco IR1101, we can meet all of those requirements with one physical device.”
Collect more data, at almost no extra cost
Using this IIoT solution, midstream operators can for the first time:
- Collect all available field data instead of just the data on a polling list. If the maintenance team requests a new type of data, the operations team can meet the request using the built-in protocol translators in Edge Intelligence. “Collecting a new type of data takes almost no extra work,” Mike said. “It makes the operations team look like heroes.”
- Collect data more frequently, helping to spot anomalies. Recording pump discharge pressure more frequently, for example, makes it easier to detect leaks. Interest in predicting (rather than responding to) equipment failure is also growing. The life of pump seals, for example, depends on both the pressure that seals experience over their lifetime and the peak pressures. “If you only collect pump pressure every 30 minutes, you probably missed the spike,” Mike explained. “If you do see the spike and replace the seal before it fails, you can prevent a very costly unexpected outage – saving far more than the cost of a new seal.”
- Protect sensitive data with end-to-end security. Security is built into the IR1101, with secure boot, VPN, certificate-based authentication, and TLS encryption.
- Give IT and OT their own interfaces so they don’t have to rely on the other team. The IT team has an interface to set up network templates to make sure device configuration is secure and consistent. Field engineers have their own interface to extract, transform, and deliver industrial data from Modbus, OPC-UA, EIP/CIP, or MQTT devices.
As Mike summed it up, “It’s finally simple to deploy a secure industrial network that makes all field data available to enterprise applications—in less time and using less bandwidth.”
Originally posted here.
TinyML focuses on optimizing machine learning (ML) workloads so that they can be processed on microcontrollers no bigger than a grain of rice and consuming only milliwatts of power.
Once again, I’m jumping up and down in excitement because I’m going to be hosting a panel discussion as part of a webinar series — Fast and Fearless: The Future of IoT Software Development — being held under the august auspices of IotCentral.io
At this event, the second of a four-part series, we will be focusing on “AI and IoT Innovation” (see also What the FAQ are AI, ANNs, ML, DL, and DNNs? and What the FAQ are the IoT, IIoT, IoHT, and AIoT?).
Panel members Karl Fezer (upper left), Wei Xiao (upper right), Nikhil Bhaskaran (lower left), and Tina Shyuan (bottom right) (Click image to see a larger version)
As we all know, the IoT is transforming the software landscape. What used to be a relatively straightforward embedded software stack has been revolutionized by the IoT, with developers now having to juggle specialized workloads, security, artificial intelligence (AI) and machine learning (ML), real-time connectivity, managing devices that have been deployed into the field… the list goes on.
In this webinar — which will be held on Tuesday 29 June 2021 from 10:00 a.m. to 11:00 a.m. CDT — I will be joined by four industry luminaries to discuss how to juggle the additional complexities that machine learning adds to IoT development, why on-device machine learning is more important now than ever, and what the combination of AI and IoT looks like for developers in the future.
The luminaries in question (and whom I will be questioning) are Karl Fezer (AI Ecosystem Evangelist at Arm), Wei Xiao (Principal Engineer, Sr. Strategic Alliances Manager at Nvidia), Nikhil Bhaskaran (Founder of Shunya OS), and Tina Shyuan (Director of Product Marketing at Qeexo).
So, what say you? Dare I hope that we will have the pleasure of your company and that you will be able to join us to (a) tease your auditory input systems with our discussions and (b) join our question-and-answer free-for-all frensy at the end? If so, may I suggest that you Register Now before all of the good virtual seats are taken, metaphorically speaking, of course.
By Sachin Kotasthane
In his book, 21 Lessons for the 21st Century, the historian Yuval Noah Harari highlights the complex challenges mankind will face on account of technological challenges intertwined with issues such as nationalism, religion, culture, and calamities. In the current industrial world hit by a worldwide pandemic, we see this complexity translate in technology, systems, organizations, and at the workplace.
While in my previous article, Humane IIoT, I discussed the people-centric strategies that enterprises need to adopt while onboarding IoT initiatives of industrial IoT in the workforce, in this article, I will share thoughts on how new-age technologies such as AI, ML, and big data, and of course, industrial IoT, can be used for effective management of complex workforce problems in a factory, thereby changing the way people work and interact, especially in this COVID-stricken world.
Workforce related problems in production can be categorized into:
- Time complexity
- Effort complexity
- Behavioral complexity
Problems categorized in either of the above have a significant impact on the workforce, resulting in a detrimental effect on the outcome—of the product or the organization. The complexity of these problems can be attributed to the fact that the workforce solutions to such issues cannot be found using just engineering or technology fixes as there is no single root-cause, rather, a combination of factors and scenarios. Let us, therefore, explore a few and seek probable workforce solutions.
Figure 1: Workforce Challenges and Proposed Strategies in Production
Addressing Time Complexity
Any workforce-related issue that has a detrimental effect on the operational time, due to contributing factors from different factory systems and processes, can be classified as a time complex problem.
Though classical paper-based schedules, lists, and punch sheets have largely been replaced with IT-systems such as MES, APS, and SRM, the increasing demands for flexibility in manufacturing operations and trends such as batch-size-one, warrant the need for new methodologies to solve these complex problems.
- Worker attendance
Anyone who has experienced, at close quarters, a typical day in the life of a factory supervisor, will be conversant with the anxiety that comes just before the start of a production shift. Not knowing who will report absent, until just before the shift starts, is one complex issue every line manager would want to get addressed. While planned absenteeism can be handled to some degree, it is the last-minute sick or emergency-pager text messages, or the transport delays, that make the planning of daily production complex.
What if there were a solution to get the count that is almost close to the confirmed hands for the shift, an hour or half, at the least, in advance? It turns out that organizations are experimenting with a combination of GPS, RFID, and employee tracking that interacts with resource planning systems, trying to automate the shift planning activity.
While some legal and privacy issues still need to be addressed, it would not be long before we see people being assigned to workplaces, even before they enter the factory floor.
During this course of time, while making sure every line manager has accurate information about the confirmed hands for the shift, it is also equally important that health and well-being of employees is monitored during this pandemic time. Use of technologies such as radar, millimeter wave sensors, etc., would ensure the live tracking of workers around the shop-floor and make sure that social distancing norms are well-observed.
- Resource mapping
While resource skill-mapping and certification are mostly HR function prerogatives, not having the right resource at the workstation during exigencies such as absenteeism or extra workload is a complex problem. Precious time is lost in locating such resources, or worst still, millions spent in overtime.
What if there were a tool that analyzed the current workload for a resource with the identified skillset code(s) and gave an accurate estimate of the resource’s availability? This could further be used by shop managers to plan manpower for a shift, keeping them as lean as possible.
Today, IT teams of OEMs are seen working with software vendors to build such analytical tools that consume data from disparate systems—such as production work orders from MES and swiping details from time systems—to create real-time job profiles. These results are fed to the HR systems to give managers the insights needed to make resource decisions within minutes.
- Worker attendance
Addressing Effort Complexity
Just as time complexities result in increased production time, problems in this category result in an increase in effort by the workforce to complete the same quantity of work. As the effort required is proportionate to the fatigue and long-term well-being of the workforce, seeking workforce solutions to reduce effort would be appreciated. Complexity arises when organizations try to create a method out-of-madness from a variety of factors such as changing workforce profiles, production sequences, logistical and process constraints, and demand fluctuations.
Thankfully, solutions for this category of problems can be found in new technologies that augment existing systems to get insights and predictions, the results of which can reduce the efforts, thereby channelizing it more productively. Add to this, the demand fluctuations in the current pandemic, having a real-time operational visibility, coupled with advanced analytics, will ensure meeting shift production targets.
- Intelligent exoskeletons
Exoskeletons, as we know, are powered bodysuits designed to safeguard and support the user in performing tasks, while increasing overall human efficiency to do the respective tasks. These are deployed in strain-inducing postures or to lift objects that would otherwise be tiring after a few repetitions. Exoskeletons are the new-age answer to reducing user fatigue in areas requiring human skill and dexterity, which otherwise would require a complex robot and cost a bomb.
However, the complexity that mars exoskeleton users is making the same suit adaptable for a variety of postures, user body types, and jobs at the same workstation. It would help if the exoskeleton could sense the user, set the posture, and adapt itself to the next operation automatically.
Taking a leaf out of Marvel’s Iron Man, who uses a suit that complements his posture that is controlled by JARVIS, manufacturers can now hope to create intelligent exoskeletons that are always connected to factory systems and user profiles. These suits will adapt and respond to assistive needs, without the need for any intervention, thereby freeing its user to work and focus completely on the main job at hand.
Given the ongoing COVID situation, it would make the life of workers and the management safe if these suits are equipped with sensors and technologies such as radar/millimeter wave to help observe social distancing, body-temperature measuring, etc.
- Highlighting likely deviations
The world over, quality teams on factory floors work with checklists that the quality inspector verifies for every product that comes at the inspection station. While this repetitive task is best suited for robots, when humans execute such repetitive tasks, especially those that involve using visual, audio, touch, and olfactory senses, mistakes and misses are bound to occur. This results in costly reworks and recalls.
Manufacturers have tried to address this complexity by carrying out rotation of manpower. But this, too, has met with limited success, given the available manpower and ever-increasing workloads.
Fortunately, predictive quality integrated with feed-forwards techniques and some smart tracking with visuals can be used to highlight the area or zone on the product that is prone to quality slips based on data captured from previous operations. The inspector can then be guided to pay more attention to these areas in the checklist.
- Intelligent exoskeletons
Addressing Behavioral Complexity
Problems of this category usually manifest as a quality issue, but the root cause can often be traced to the workforce behavior or profile. Traditionally, organizations have addressed such problems through experienced supervisors, who as people managers were expected to read these signs, anticipate and align the manpower.
However, with constantly changing manpower and product variants, these are now complex new-age problems requiring new-age solutions.
- Heat-mapping workload
Time and motion studies at the workplace map the user movements around the machine with the time each activity takes for completion, matching the available cycle-time, either by work distribution or by increasing the manpower at that station. Time-consuming and cumbersome as it is, the complexity increases when workload balancing is to be done for teams working on a single product at the workstation. Movements of multiple resources during different sequences are difficult to track, and the different users cannot be expected to follow the same footsteps every time.
Solving this issue needs a solution that will monitor human motion unobtrusively, link those to the product work content at the workstation, generate recommendations to balance the workload and even out the ‘congestion.’ New industrial applications such as short-range radar and visual feeds can be used to create heat maps of the workforce as they work on the product. This can be superimposed on the digital twin of the process to identify the zone where there is ‘congestion.’ This can be fed to the line-planning function to implement corrective measures such as work distribution or partial outsourcing of the operation.
- Aging workforce (loss of tribal knowledge)
With new technology coming to the shop-floor, skills of the current workforce get outdated quickly. Also, with any new hire comes the critical task of training and knowledge sharing from experienced hands. As organizations already face a shortage of manpower, releasing more hands to impart training to a larger workforce audience, possibly at different locations, becomes an even more daunting task.
Fully realizing the difficulties and reluctance to document, organizations are increasingly adopting AR-based workforce trainings that map to relevant learning and memory needs. These AR solutions capture the minutest of the actions executed by the expert on the shop-floor and can be played back by the novice in-situ as a step-by-step guide. Such tools simplify the knowledge transfer process and also increase worker productivity while reducing costs.
Further, in extraordinary situations such as the one we face at present, technologies such as AR offer solutions for effective and personalized support to field personnel, without the need to fly in specialists at multiple sites. This helps keep them safe, and accessible, still.
- Heat-mapping workload
Key takeaways and Actionable Insights
The shape of the future workforce will be the result of complex, changing, and competing forces. Technology, globalization, demographics, social values, and the changing personal expectations of the workforce will continue to transform and disrupt the way businesses operate, increasing the complexity and radically changing where, and when of future workforce, and how work is done. While the need to constantly reskill and upskill the workforce will be humongous, using new-age techniques and technologies to enhance the effectiveness and efficiency of the existing workforce will come to the spotlight.
Figure 2: The Future IIoT Workforce
Organizations will increasingly be required to:
- Deploy data farming to dive deep and extract vast amounts of information and process insights embedded in production systems. Tapping into large reservoirs of ‘tribal knowledge’ and digitizing it for ingestion to data lakes is another task that organizations will have to consider.
- Augment existing operations systems such as SCADA, DCS, MES, CMMS with new technology digital platforms, AI, AR/VR, big data, and machine learning to underpin and grow the world of work. While there will be no dearth of resources in one or more of the new technologies, organizations will need to ‘acqui-hire’ talent and intellectual property using a specialist, to integrate with existing systems and gain meaningful actionable insights.
- Address privacy and data security concerns of the workforce, through the smart use of technologies such as radar and video feeds.
Nonetheless, digital enablement will need to be optimally used to tackle the new normal that the COVID pandemic has set forth in manufacturing—fluctuating demands, modular and flexible assembly lines, reduced workforce, etc.
Originally posted here.
Flowchart of IoT in Mining
by Vaishali Ramesh
Introduction – Internet of Things in Mining
The Internet of things (IoT) is the extension of Internet connectivity into physical devices and everyday objects. Embedded with electronics, Internet connectivity, and other forms of hardware; these devices can communicate and interact with others over the Internet, and they can be remotely monitored and controlled. In the mining industry, IoT is used as a means of achieving cost and productivity optimization, improving safety measures and developing their artificial intelligence needs.
IoT in the Mining Industry
Considering the numerous incentives it brings, many large mining companies are planning and evaluating ways to start their digital journey and digitalization in mining industry to manage day-to-day mining operations. For instance:
- Cost optimization & improved productivity through the implementation of sensors on mining equipment and systems that monitor the equipment and its performance. Mining companies are using these large chunks of data – 'big data' to discover more cost-efficient ways of running operations and also reduce overall operational downtime.
- Ensure the safety of people and equipment by monitoring ventilation and toxicity levels inside underground mines with the help of IoT on a real-time basis. It enables faster and more efficient evacuations or safety drills.
- Moving from preventive to predictive maintenance
- Improved and fast-decision making The mining industry faces emergencies almost every hour with a high degree of unpredictability. IoT helps in balancing situations and in making the right decisions in situations where several aspects will be active at the same time to shift everyday operations to algorithms.
IoT & Artificial Intelligence (AI) application in Mining industry
Another benefit of IoT in the mining industry is its role as the underlying system facilitating the use of Artificial Intelligence (AI). From exploration to processing and transportation, AI enhances the power of IoT solutions as a means of streamlining operations, reducing costs, and improving safety within the mining industry.
Using vast amounts of data inputs, such as drilling reports and geological surveys, AI and machine learning can make predictions and provide recommendations on exploration, resulting in a more efficient process with higher-yield results.
AI-powered predictive models also enable mining companies to improve their metals processing methods through more accurate and less environmentally damaging techniques. AI can be used for the automation of trucks and drills, which offers significant cost and safety benefits.
Challenges for IoT in Mining
Although there are benefits of IoT in the mining industry, implementation of IoT in mining operations has faced many challenges in the past.
- Limited or unreliable connectivity especially in underground mine sites
- Remote locations may struggle to pick up 3G/4G signals
- Declining ore grade has increased the requirements to dig deeper in many mines, which may increase hindrances in the rollout of IoT systems
Mining companies have overcome the challenge of connectivity by implementing more reliable connectivity methods and data-processing strategies to collect, transfer and present mission critical data for analysis. Satellite communications can play a critical role in transferring data back to control centers to provide a complete picture of mission critical metrics. Mining companies worked with trusted IoT satellite connectivity specialists such as ‘Inmarsat’ and their partner eco-systems to ensure they extracted and analyzed their data effectively.
Cybersecurity will be another major challenge for IoT-powered mines over the coming years
As mining operations become more connected, they will also become more vulnerable to hacking, which will require additional investment into security systems.
Following a data breach at Goldcorp in 2016, that disproved the previous industry mentality that miners are not typically targets, 10 mining companies established the Mining and Metals Information Sharing and Analysis Centre (MM-ISAC) to share cyber threats among peers in April 2017.
In March 2019, one of the largest aluminum producers in the world, Norsk Hydro, suffered an extensive cyber-attack, which led to the company isolating all plants and operations as well as switching to manual operations and procedures. Several of its plants suffered temporary production stoppages as a result. Mining companies have realized the importance of digital security and are investing in new security technologies.
Digitalization of Mining Industry - Road Ahead
Many mining companies have realized the benefits of digitalization in their mines and have taken steps to implement them. There are four themes that are expected to be central to the digitalization of the mining industry over the next decade are listed below:
The above graph demonstrates the complexity of each digital technology and its implementation period for the widespread adoption of that technology. There are various factors, such as the complexity and scalability of the technologies involved in the adoption rate for specific technologies and for the overall digital transformation of the mining industry.
The world can expect to witness prominent developments from the mining industry to make it more sustainable. There are some unfavorable impacts of mining on communities, ecosystems, and other surroundings as well. With the intention to minimize them, the power of data is being harnessed through different IoT statements. Overall, IoT helps the mining industry shift towards resource extraction, keeping in mind a particular time frame and footprint that is essential.
Originally posted here.
In this blog, we’ll discuss how users of Edge Impulse and Nordic can actuate and stream classification results over BLE using Nordic’s UART Service (NUS). This makes it easy to integrate embedded machine learning into your next generation IoT applications. Seamless integration with nRF Cloud is also possible since nRF Cloud has native support for a BLE terminal.
We’ve extended the Edge Impulse example functionality already available for the nRF52840 DK and nRF5340 DK by adding the abilities to actuate and stream classification outputs. The extended example is available for download on github, and offers a uniform experience on both hardware platforms.
Using nRF Toolbox
After following the instructions in the example’s readme, download the nRF Toolbox mobile application (available on both iOS and Android) and connect to the nRF52840 DK or the nRF5340 DK that will be discovered as “Edge Impulse”. Once connected, set up the interface as follows so that you can get information about the device, available sensors, and start/stop the inferencing process. Save the preset configuration so that you can load it again for future use. Fill out the text of the various commands to use the same convention as what is used for the Edge Impulse AT command set. For example, sending AT+RUNIMPULSE starts the inferencing process on the device.
Figure 1. Setting up the Edge Impulse AT Command set
Once the appropriate AT command set mapping to an icon has been done, hit the appropriate icon. Hitting the ‘play’ button cause the device to start acquiring data and perform inference every couple of seconds. The results can be viewed in the “Logs” menu as shown below.
Figure 2. Classification Output over BLE in the Logs View
Using nRF Cloud
Using the nRF Connect for Cloud mobile app for iOS and Android, you can turn your smartphone into a BLE gateway. This allows users to easily connect their BLE NUS devices running Edge Impulse to the nRF Cloud as an easy way to send the inferencing conclusions to the cloud. It’s as easy as setting up the BLE gateway through the app, connecting to the “Edge Impulse” device and watching the same results being displayed in the “Terminal over BLE” window shown below!
Figure 3. Classification Output Shown in nRF Cloud
Summary
Edge Impulse is supercharging IoT with embedded machine learning and we’ve discussed a couple of ways you can easily send conclusions to either the smartphone or to the cloud by leveraging the Nordic UART Service. We look forward to seeing how you’ll leverage Edge Impulse, Nordic and BLE to create your next gen IoT application.
Article originally written for the Edge Impulse blog by Zin Thein Kyaw, Senior User Success Engineer at Edge Impulse.